Artificial Intelligence (AI) is transforming industries, influencing decision-making processes, and significantly impacting the way societies function. As AI systems become increasingly integrated into our daily lives, the ethical implications of their use come to the forefront. One of the central concerns in the ethical landscape of AI is the issue of bias and fairness in algorithms. This comprehensive article delves into the complexities of ethical considerations in AI, focusing on the challenges posed by bias and the ongoing efforts to ensure fairness in algorithmic decision-making.
Understanding Bias in AI:
Bias in AI refers to the presence of systematic and unfair preferences or prejudices in the outcomes produced by algorithms. These biases can emerge from various sources, including biased training data, flawed algorithm design, or the influence of the societal context in which the AI system operates. Understanding the different forms of bias is crucial for developing strategies to address and mitigate its impact.
Data Bias:
Data used to train AI models often reflects historical biases present in society. If the training data is biased, the AI model may perpetuate or even exacerbate those biases. For example, biased data in facial recognition systems can result in inaccurate or unfair outcomes, particularly for underrepresented groups.
Algorithmic Bias:
Algorithmic bias occurs when the design and functionality of the algorithm itself introduce or reinforce biases. This can happen if the features considered by the algorithm are selected in a way that unintentionally discriminates against certain groups. An algorithm trained on biased data might learn patterns that reinforce existing stereotypes.
Outcome Bias:
The outcomes produced by AI systems may disproportionately favor or disadvantage specific groups, even when the algorithm itself is unbiased. This form of bias can arise due to a complex interplay of factors, including the inherent complexity of the system, the choice of evaluation metrics, and the context in which the AI is deployed.
Challenges in Addressing Bias:
Addressing bias in AI is a multifaceted challenge that involves technological, ethical, and regulatory considerations. Several key challenges impede the progress in achieving fairness and mitigating bias in AI systems.
Lack of Diversity in Development Teams:
The composition of development teams working on AI projects can influence the identification and mitigation of bias. Homogeneous teams may inadvertently overlook biases that affect diverse populations. Ensuring diversity in AI development teams is crucial for uncovering and addressing potential biases.
Complexity of AI Systems:
AI models, especially deep learning models, can be highly complex and opaque. Understanding how these models arrive at specific decisions is a significant challenge. The lack of interpretability makes it difficult to identify and rectify biases effectively.
Dynamic Nature of Bias:
Bias in AI systems is not static; it can evolve over time based on changes in the data distribution or societal norms. Continuous monitoring and adaptation are necessary to address new forms of bias that may emerge as the system interacts with the real world.
Ethical Considerations:
Determining what is considered fair or ethical can vary across cultures and societies. Establishing universally accepted ethical guidelines for AI is challenging, and the interpretation of fairness may differ among stakeholders.
Strategies for Mitigating Bias and Ensuring Fairness:
Efforts to address bias and ensure fairness in AI involve a combination of technical solutions, ethical frameworks, and regulatory measures. The goal is not only to rectify biases in existing systems but also to prevent biases from being perpetuated in future AI developments.
Diverse and Inclusive Data Collection:
Ensuring diversity and representativeness in training data is fundamental to mitigating bias. AI developers must carefully curate datasets that include a broad range of demographic, ethnic, and socio-economic factors, preventing the reinforcement of existing biases.
Explainable AI (XAI):
Developing AI systems that are explainable and interpretable is crucial for understanding how decisions are made. Explainable AI (XAI) methods aim to provide insights into the inner workings of complex models, allowing developers and end-users to grasp the factors influencing outcomes.
Algorithmic Audits:
Conducting regular audits of AI algorithms helps identify and rectify biases. Audits involve evaluating the system’s outputs against predefined fairness criteria and addressing discrepancies. This iterative process helps refine algorithms over time.
Ethics by Design:
Integrating ethical considerations into the design and development phases of AI projects is known as ethics by design. This involves anticipating potential biases, assessing the social impact of AI applications, and incorporating ethical principles into the decision-making processes of the system.
Regulatory Frameworks:
Governments and regulatory bodies are increasingly recognizing the need to establish frameworks that govern the ethical use of AI. Regulations can set standards for transparency, accountability, and fairness in AI systems, providing a legal basis for addressing biases.
Bias Mitigation Techniques:
Several techniques can be employed to mitigate bias in AI models, including re-sampling techniques, adversarial training, and the use of fairness-aware algorithms. These methods aim to correct imbalances in training data and reduce biases in the model’s decision-making process.
Case Studies: Real-World Examples of Bias in AI:
Examining real-world instances where bias in AI has led to significant consequences provides insights into the challenges and repercussions of algorithmic bias.
Facial Recognition Bias:
Facial recognition systems have demonstrated biases, particularly in their accuracy across different demographic groups. Studies have shown that these systems can be less accurate for women, people with darker skin tones, and individuals from certain ethnic backgrounds, leading to concerns about misidentification and discrimination.
Predictive Policing:
AI algorithms used in predictive policing have faced scrutiny for perpetuating biases present in historical crime data. If the training data reflects existing biases in law enforcement practices, the algorithm may recommend interventions that disproportionately target certain communities.
Credit Scoring Algorithms:
Credit scoring algorithms have been criticized for perpetuating economic and racial biases. If historical lending data reflects discriminatory practices, AI models trained on this data may inadvertently disadvantage certain demographic groups when determining creditworthiness.
The Role of Stakeholders in Ensuring Ethical AI:
Addressing bias and ensuring fairness in AI is a collective responsibility that involves multiple stakeholders, including developers, policymakers, industry leaders, and the general public.
Developers and Researchers:
AI developers and researchers play a central role in identifying and mitigating biases in AI systems. Emphasizing diversity in development teams, conducting thorough testing, and incorporating ethical considerations into the development process are essential steps.
Policymakers and Regulators:
Governments and regulatory bodies play a crucial role in establishing clear guidelines and regulations for the ethical use of AI. This includes defining standards for transparency, accountability, and fairness, and enforcing consequences for violations.
Industry Leaders:
Companies at the forefront of AI development must lead by example. Implementing ethical frameworks, fostering diversity in the workplace, and prioritizing transparency in AI systems contribute to a culture of responsible AI development.
Educational Institutions:
Educational institutions have a responsibility to equip future AI professionals with a strong understanding of ethical considerations. Integrating ethics into AI education ensures that the next generation of developers is well-prepared to navigate the ethical challenges of AI.
Civil Society and Advocacy Groups:
Civil society organizations and advocacy groups play a vital role in raising awareness about AI bias and advocating for ethical practices. These groups contribute to public discourse, hold stakeholders accountable, and push for policies that prioritize fairness and equity.
The Future of Ethical AI:
As the field of AI continues to advance, the quest for ethical AI is an ongoing journey. The future of ethical AI involves continuous innovation in mitigating bias, refining fairness standards, and adapting to the evolving landscape of technology and society.
Explainability and Transparency:
Advancements in explainable AI will contribute to greater transparency in AI systems. Innovations in model interpretability will empower users to understand and trust the decisions made by AI algorithms.
AI for Social Good:
The use of AI for social good initiatives is gaining traction. AI applications that address societal challenges, promote inclusivity, and contribute to global well-being are essential for fostering a positive impact.
Global Collaboration:
Given the global nature of AI, international collaboration is crucial. The development of ethical AI requires cooperation among nations, sharing best practices, and establishing global standards to ensure a consistent approach to fairness and bias mitigation.
Empowering Users:
Empowering end-users with control over their data and the decisions made by AI systems is pivotal. Providing individuals with the ability to understand, contest, or opt-out of algorithmic decisions enhances user agency and accountability.
Ongoing Research and Innovation:
Continuous research and innovation are essential to stay ahead of emerging challenges in AI ethics. Interdisciplinary collaboration between computer scientists, ethicists, social scientists, and policymakers will drive the development of ethical frameworks and solutions.
Conclusion:
Addressing bias and ensuring fairness in AI is not a singular task but a complex, ongoing process that requires the concerted efforts of multiple stakeholders. As AI becomes an integral part of our lives, the ethical considerations surrounding its use become increasingly critical. By adopting a proactive approach, embracing diversity, implementing robust regulatory frameworks, and prioritizing transparency, the global community can navigate the ethical complexities of AI and shape a future where AI technologies contribute positively to society. The pursuit of ethical AI is not just a technological imperative; it is a moral obligation to ensure that the benefits of AI are accessible, fair, and equitable for all.
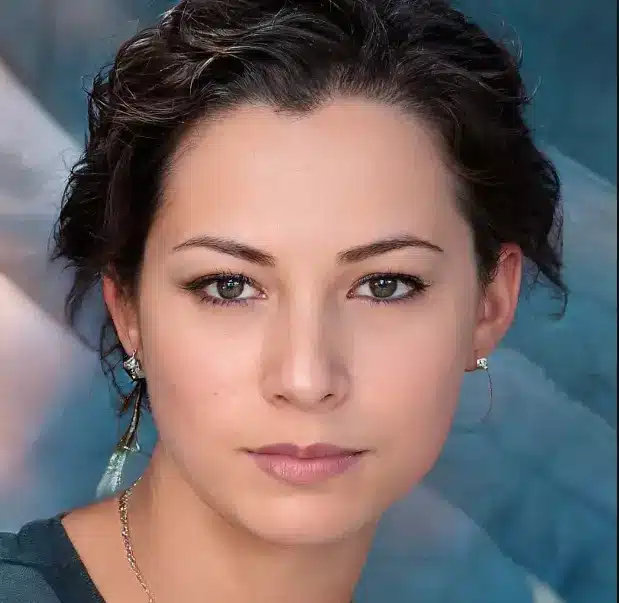
Meet Catherine Mellor, a tech enthusiast and the creative mind behind our AI-focused blogs. Catherine is on a mission to unravel the mysteries of artificial intelligence and make this cutting-edge technology accessible to all. With a background in computer science and a passion for the limitless possibilities AI presents, she crafts insightful blogs that explore the intersections of AI with daily life, business, and the future.
Join Catherine on a journey through the ever-evolving landscape of artificial intelligence as she breaks down complex concepts, shares the latest advancements, and sparks conversations about the ethical and societal implications of AI.
Connect on email: [email protected]